The Evolution of Intelligent Search and Where It's Going
The volume of information collected for a smart city continues to increase on a daily basis, and this includes video data. This growth is due to several factors. First of all, devices for generating video content (such as video cameras and recorders) are getting cheaper: the price of video cameras has decreased by about five times over the past seven years.
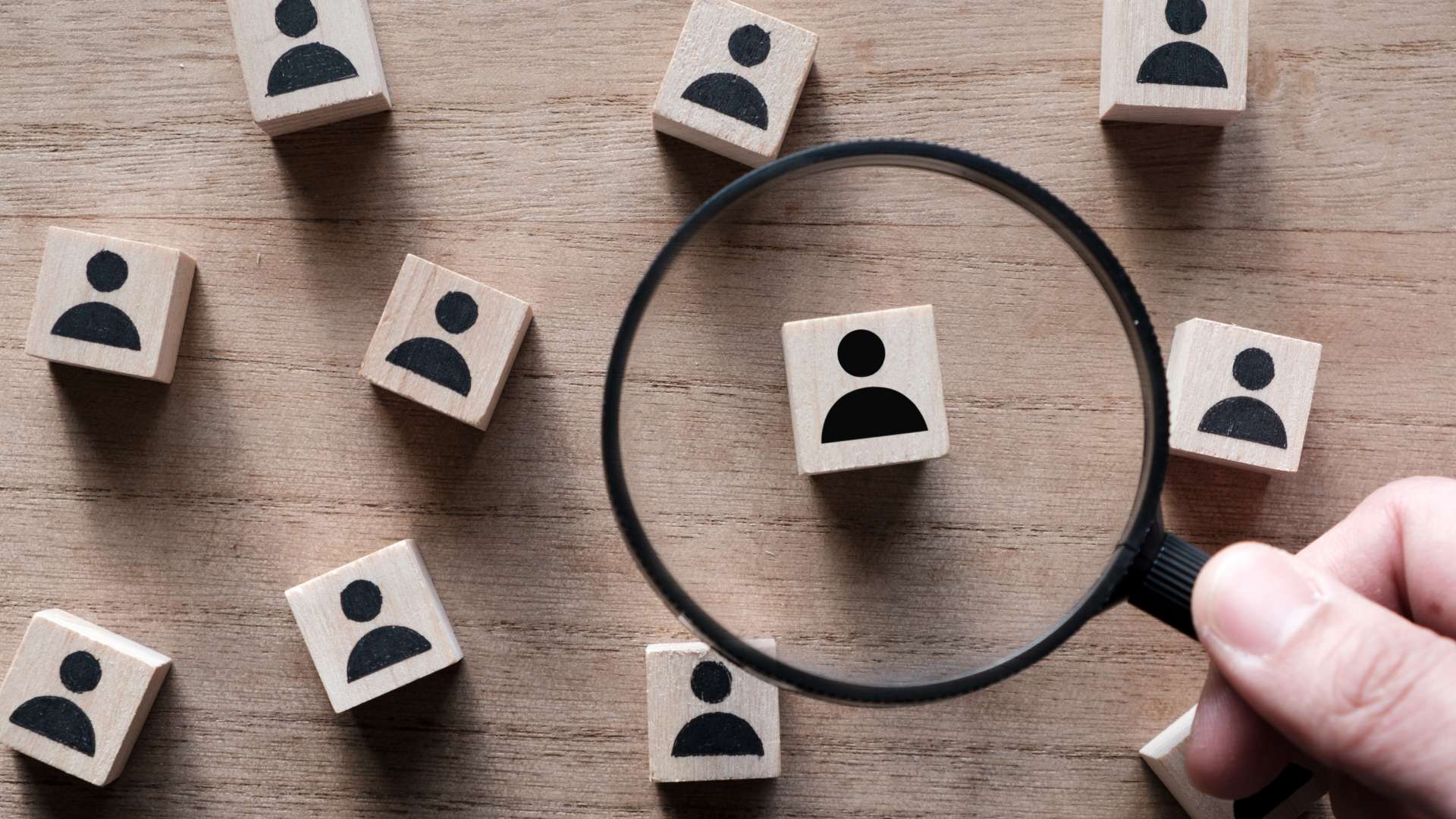
While cameras have become more affordable, the amount of content they can accumulate has grown. Data storage has also gotten less expensive, while gaining in speed and volume. The cost of transmitting a unit of information has also been reduced by more than five times. The result has been a dramatic increase in the scale of Safe City pilot projects (the minimum technical components): where previously a project might have included 10 to 20 cameras, now it starts out with 100 to 500 cameras. So the biggest issue today is what to do with the data that is collected.
Googleless Internet
The Internet (called ARPANET at the time) was born in 1969. However, the first search service wasn't available until 1990. Can you imagine how the Internet existed without search engines for almost 20 years? Users were comfortable with this, because there wasn't that much information available inside this framework and people were able to share it directly, through the print media, and so on. Once search engines came along, it became impossible to think of living without them.
Modern CCTV systems can be compared to a "Googleless Internet". Obviously, this is inefficient and inconvenient. With 10–20 cameras, you can search manually to find the information you need, if you remember which scenes are covered by cameras and you have an idea of who or what to look for. But when you have 500 cameras, you can't get by without search technology.
A Convenient Interface: The Beginnings of a Search Tool
The first technologies that formed the seeds of video search were related to developing functional and user-friendly interfaces. Even showing the exact date and time on the footage was enough to allow searching based on these criteria. It became clear that there was a demand for tools that would make the operator's job easier. And the first ideas were again related to interfaces that make it convenient and easy to find events.
One of these is to search for a trigger event by approximation (an example is when the video shows that a car has disappeared from its parking spot). To do this, a lengthy section of the archive is split into several equal parts. For example, a 12-hour video is divided into 12 parts of one hour each, and the screen shows a preview of each one (the first few frames of each section). This lets you identify which section of video still had the object in it, and in which section it went missing, which means that the trigger event occurred in that time range.
By incrementally dividing each section into smaller ones, you can ultimately determine the time of the event up to the second. In the case of a 12-hour recording, you'll only need four clicks to find the event. This seems like intelligent search, doesn't it? But it's actually just a good interface feature.
LPR Search: The First Intelligent Technology
Despite the efficiency of searching by fragments, it doesn't meet every need. For example, you can't see who entered a particular area, or find all the cars that were in the camera's field of view.
The first truly intelligent search was license plate recognition technology. The algorithms for recognizing a license plate and transforming the image into text have actually existed since analog systems. These algorithms could detect all of the license plates that showed up in a frame, and then search those records like searching through a notebook. (With recent developments in recognition algorithms, we now have similar technologies to search for faces.)
So the idea of describing a scene in advance in order to then use this data for searching was already floating around. It was only lacking the means to describe the scenes, and these emerged over time – technologies for describing faces in geometric terms and tracking the behavior of objects. The combination of all these tools has made it possible to generate metadata that defines the scene and everything in it with a high degree of precision.
If Search Isn't Instant, It's Pointless
At this point, a serious question arose: where should the metadata be stored? The market didn't offer effective tools that could allow both storing the data and searching the geometric description of a scene. Normal relational databases are designed for structured information that can be indexed (fields like "height, weight, chest size, criminal record, security clearance"). But geometric data is a chaotic array of digits that are generated extremely quickly, because the objects in the frame are constantly moving. At the same time, it is important to store as much data as possible (object coordinates, size, color, and so on), because the more information about the object is available, the easier it will be to find it. This type of data can be stored in a relational database, but the search will be very slow.
Another problem was that in describing the scene, there was no way to filter the data into useful, useless, and bad information. Everything had to be stored, and the scene description had to be as detailed as possible. There was no way to know whether an event is useful or detrimental until the time of the search. So when searching for a person in the bushes, wind rustling in the bushes creates false alarms, which is bad information. But if you're looking for information on whether the wind was blowing at a certain point, someone in the bushes becomes bad information, while the rustling bushes are now useful because they are moving from the wind.
During a search, the ratio of useful information to all other information depends mainly on how specific the search criteria is. The most effective criteria are almost impossible to set at the first try, so you always need to change and adjust them (make a line shorter or longer, change the color gradient, expand or narrow the area of the search frame, and so on). The only way to do this is to experiment, so the search is efficient only if the results are instant and the search criteria can be adjusted immediately. If you have to wait at all, there isn't any point to it, since the user will simply give up after 2 or 3 attempts. The analogy with the Internet is also appropriate here: when you get search results quickly, you can adapt your search query with different keywords. If you had to wait for five minutes after each search, you wouldn't have the patience to find the right keywords and get the results. That's why instant search is so important: it's what makes the system effective.
Returning to the problem of storing the metadata, up until a certain point, there weren't any existing storage systems that allowed instant retrieval of search results. To solve this problem, companies invested in expensive research and development, which resulted in unique mediums optimized for storing geometric data, so now they have the technology to quickly extract information. This is how they are able to make their search systems truly effective and can fully meet the challenges of urban and regional security systems encompassing thousands and tens of thousands of cameras.
The Future of Intelligent Search
Search engines are improving every day, but this development path will eventually reach its limit, since the only aspect being developed is the tools for generating qualitative scene descriptions. More of them may be developed, and they may become broader, more precise, and so on. But the future clearly belongs to systems that can not only describe the scene, but also interpret what has happened in the scene, meaning they can add semantic markers.
Right now, the operator searches for some movement in the scene, which is just the movement of abstract objects as far as the software is concerned. At best, it is classified as "person, car, or crowd of people," but it's rather arbitrary. What's needed is for the VMS to start to understand what the objects in the scene are doing. For example, consider a situation in which a man scratched a car. It would be very nice if the VMS started to recognize that it wasn't that Object 1 got close to Object 2 and then they moved apart, but that it was specifically a person who scratched a car, as opposed to opening it or looking in the side mirror. When the system can make a meaningful assessment of what is happening, it will be possible to evaluate the behavior of objects in the frame and identify "suspicious" activity and so on. Naturally, this will be an astounding leap forward in search quality, and it's going to be propelled by the semantic description of the scene.
In coming years, most efforts will be focused on creating tools that make it easier and faster for the operator to find the right fragment of video archive based on a qualitative description. It's possible that stereoscopic vision will help by adding another dimension, which would make it possible to see how far an object is from the camera's view point. This would allow the operator to search for objects by degree of distance or the real geometry of the object's size (relative dimensions are currently used).
We are all still at the beginning of this journey. 2016 showed an obvious trend, as people started to realize the importance of search and became interested in the tools available. For Safe City projects, it is essential to have instant multi-camera search for faces, LPR, and any other objects and events within the tremendous volumes of video recordings. So we are likely to see some inevitable changes soon: any video surveillance system, regardless of its scale, will simply have to incorporate search tools such as those described in this article or something similar. The era of the "Googleless Internet" is on its way out, never to return.
wróć do bloga